Is Asset Price a Good Metric for Predictive Maintenance Feasibility?
Jun 7, 2024
Predictive MaintenanceIn the world of industrial maintenance, the pressure to optimize is constant. We're always looking for smarter ways to prevent costly downtime and extend the life of our critical equipment. Predictive maintenance software, like ours at Factory AI, promises a proactive approach. We've previously discussed how to calculate its potential benefits and quick back-of-the-napkin methods to see if it's a fit. Now, it's time to tackle a persistent myth: that the initial cost of an asset should be the primary driver in deciding whether to include it in your predictive maintenance program. Let's explore why that thinking might be holding you back.
Given that reliability leaders are focused (rightly so) on ensuring every dollar invested offers a meaningful payback, we’re writing a series of articles to help you understand how you can evaluate predictive maintenance software, such as ours at Factory AI.
In the past, we’ve discussed three simple steps to calculate whether smart, continuous condition monitoring software with intelligence could help. We've also shown how you can use the simple failure history method as a quick back-of-the-napkin trick to decide whether you should explore predictive maintenance further.
In this article, we want to address a common misconception about evaluating predictive maintenance.
First, Let’s Talk About Asset Criticality
We are all familiar with asset criticality models. To classify assets by criticality, we essentially ask, “What is the asset’s effect on production output?” You would then consider safety criticality, production impact, repair time/cost, and environmental impact to help you make your decision.
There are more layers to this, but in reality, most of the sites we work with end up using a three-tier approach where they classify assets as either:
- Critical
- Semi-Critical or Essential
- Non-Critical or General Purpose
An Example Asset-Criticality Document (Source: Fluke)
Why the Price of the Asset Isn’t a Great Proxy for Perceived Value
When you look at a site’s asset criticality document, you will notice that criticality and asset price aren’t directly correlated.
You will likely find critical assets that are cheaper than some essential or general-purpose assets. This is typically true for assets like pumps that are needed to power nearly all the production lines and may not be as technically expensive as some cooling tower fans (if you consider the control unit and all bells and whistles).
Where the True Value of Predictive Maintenance Lies
Asset criticality is a better proxy for the potential value you would get from using predictive maintenance software, rather than simply the price of an asset.
This is because the real pain of unplanned downtime isn’t so much related to the value of the asset causing the downtime. The pain is more associated with the impact of production losses due to the downtime.
In their “Predictive Maintenance Market: 5 Highlights for 2024 and Beyond” report, IoT Analytics writes that “the median unplanned downtime cost across 11 industries is approximately $125,000 per hour.”
For many of our customers, this figure is overblown. Their true hourly downtime cost is more around the $10,000 mark. Still, if a small motor on a conveyor goes down and halts production for two hours, that’s $20,000 out the window. Spending $750 per year to monitor this asset (which is the type of asset we have a history of accurately predicting failures for) would make sense. This is true even if the value of such a motor is only $4,000.
A view of asset pages in the Factory Ai Predictive Maintenance Platform
Conclusion
With this simple example, you can see why the price of an asset is not a great proxy for determining whether it makes sense to include such an asset as part of your predictive maintenance program.
Moreover, companies that offer predictive maintenance software platforms, such as Factory AI, should be able to provide you with multiple smart monitoring strategies. For some sites, it makes sense to instrument highly critical assets with more, higher-quality sensors to better capture diagnostic information when parameters start to deviate from the normal healthy range. For more general-purpose assets that are smaller and have simple operating profiles, a very simple setup might work just fine.
If you’d like to stress test your predictive maintenance plan with someone who’s helped multiple food and beverage sites implement them successfully, even if simply for advice, we’re here to help.
Speak soon.
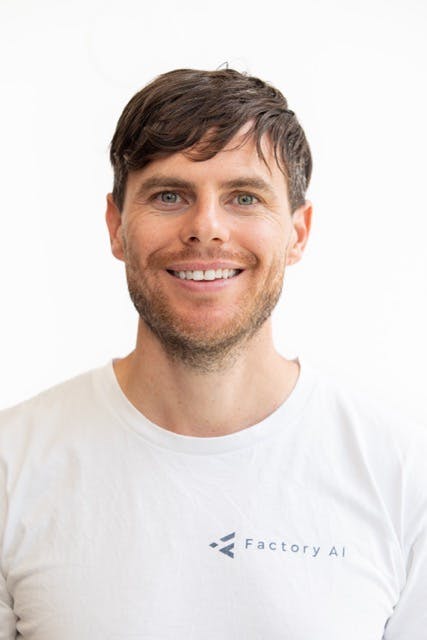
JP Picard
Jean-Philippe Picard is the CEO and Co-Founder of Factory AI. As a positive, transparent, and confident business development leader, he is passionate about helping industrial sites achieve tangible results by focusing on clean, accurate data and prioritizing quick wins. Jean-Philippe has a keen interest in how maintenance strategies evolve and believes in the importance of aligning current practices with a site’s future needs, especially with the increasing accessibility of predictive maintenance and AI. He understands the challenges of implementing new technologies, including addressing potential skills and culture gaps within organizations. Jean-Philippe is based in Sydney, New South Wales, Australia.